Note
Go to the end to download the full example code.
Quick start#
From your Python code, create and load a skore Project
:
import skore
my_project = skore.open("my_project", create=True)
This will create a skore project directory named quick_start.skore
in your
current working directory. Note that overwrite=True
will overwrite any pre-existing
project with the same path (which you might not want to do that depending on your use
case).
Evaluate your model using skore’s CrossValidationReport
:
from sklearn.datasets import make_classification
from sklearn.linear_model import LogisticRegression
from skore import CrossValidationReport
X, y = make_classification(n_classes=2, n_samples=100_000, n_informative=4)
clf = LogisticRegression()
cv_report = CrossValidationReport(clf, X, y)
Processing cross-validation ━━━━━━━━━━━━━━━━━━━━━━━━━━━━━━━━━━━━━━━━ 100%
for LogisticRegression
Display the help tree to see all the insights that are available to you (given that you are doing binary classification):
╭─────────────────── Tools to diagnose estimator LogisticRegression ───────────────────╮
│ report │
│ ├── .metrics │
│ │ ├── .accuracy(...) (↗︎) - Compute the accuracy score. │
│ │ ├── .brier_score(...) (↘︎) - Compute the Brier score. │
│ │ ├── .log_loss(...) (↘︎) - Compute the log loss. │
│ │ ├── .precision(...) (↗︎) - Compute the precision score. │
│ │ ├── .recall(...) (↗︎) - Compute the recall score. │
│ │ ├── .roc_auc(...) (↗︎) - Compute the ROC AUC score. │
│ │ ├── .custom_metric(...) - Compute a custom metric. │
│ │ ├── .report_metrics(...) - Report a set of metrics for our estimator. │
│ │ └── .plot │
│ │ ├── .precision_recall(...) - Plot the precision-recall curve. │
│ │ └── .roc(...) - Plot the ROC curve. │
│ ├── .cache_predictions(...) - Cache the predictions for sub-estimators │
│ │ reports. │
│ ├── .clear_cache(...) - Clear the cache. │
│ └── Attributes │
│ ├── .X │
│ ├── .y │
│ ├── .estimator_ │
│ ├── .estimator_name_ │
│ ├── .estimator_reports_ │
│ └── .n_jobs │
│ │
│ │
│ Legend: │
│ (↗︎) higher is better (↘︎) lower is better │
╰──────────────────────────────────────────────────────────────────────────────────────╯
Display the report metrics that was computed for you:
df_cv_report_metrics = cv_report.metrics.report_metrics()
df_cv_report_metrics
Compute metric for each split ━━━━━━━━━━━━━━━━━━━━━━━━━━━━━━━━━━━━━━━━ 100%
Display the ROC curve that was generated for you:
import matplotlib.pyplot as plt
roc_plot = cv_report.metrics.plot.roc()
roc_plot
plt.tight_layout()
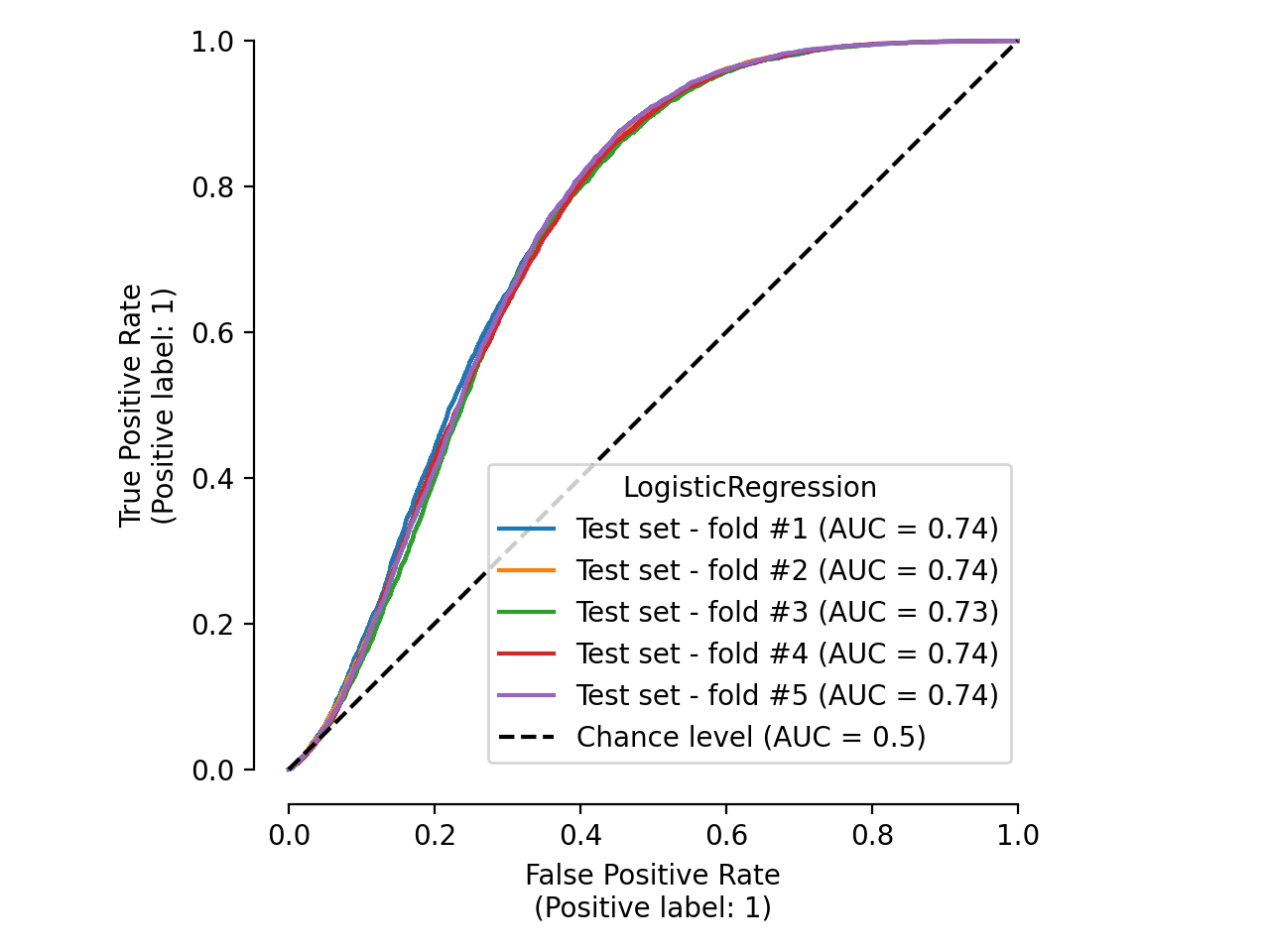
Computing predictions for display ━━━━━━━━━━━━━━━━━━━━━━━━━━━━━━━━━━━━━━━━ 100%
Store the results in the skore project for safe-keeping:
my_project.put("df_cv_report_metrics", df_cv_report_metrics)
my_project.put("roc_plot", roc_plot)
What’s next?
For a more in-depth guide, see our Skore: getting started page!
Cleanup the project#
Let’s clear the skore project (to avoid any conflict with other documentation examples).
Total running time of the script: (0 minutes 1.961 seconds)